Mathematical Basis - Squashing Function
2018-08-11
Machine-Learning
444
This article is about some squashing functions of deep learning, including Softmax Function, Sigmoid Function, and Hyperbolic Functions. All of these three functions are used to squash value to a certain range.
Softmax Function
Softmax Function: A generalization of the logistic function that "squashes" a K-dimensional vector z of arbitrary real values to a K-dimensional vector of real values, where each entry is in the range (0, 1], and all the entries add up to 1.
In probability theory, the output of the softmax function can be used to represent a categorical distribution - that is, a probability distribution over K different possible outcomes.
The softmax function is the gradient of the LogSumExp function.
LogSumExp Function
LogSumExp Function: The LogSumExp(LSE) function is a smooth approximation to the maximum function.
( stands for the natural logarithm function, i.e. the logarithm to the base e.)
When directly encountered, LSE can be well-approximated by :
Sigmoid
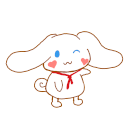