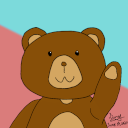
Donny
Hello, welcome to... Wait, stop clicking my avatar!
Search is dying, use ChatGPT plugins.
2023-03-25
Articles
469
Many people are saying that AI powered search will be the future. It is not. With the announcement of ChatGPT plugins, if you are still thinking that search will be important in the future, then you are still in the old mindset.
Search is only a bridge between the old fashion web interface, and the new AI powered language interface. Search gives AI access to the traditional web. But what will really empower AI is the plugin based language interface. One important component that will play a key role is the information retrieval plugin open sourced by OpenAI.
Imagine you are a website owner, you could sit still and let AI powered search to crawl and understand your website. Or you can provide your own plugin that makes use of the information retrieval plugin provided by OpenAI, to serve your well-indexed contents when ChatGPT decided to use your plugin. The former one is passive, and the later one is active. Search is non-deterministic,
Tech Dec 2022
2022-12-10
Tech
748
12月10日 土曜
前は日記に置いてるが、やはりテックのものを別記事にまとめます。
Stable Diffusion
Stable Diffusionを安定で高質の絵を出力させるには呪文が必要らしい。この文章を参考しました。最初はプロンプトだけを使ってるが、顔や体が歪んでしまいがち。ネガティブプロンプトがあることを思い出して、試しに入れてみたら歪みが大分治まった。
現時点で一番いいプロンプト。
--prompt "${normal_prompt_content} \
with fantastic lighting fantastic composition \
in high production quality" \
--negative-prompt "twisted face twisted body closeup view"
一部の出力。
銃を持つ学校制服を着てる少女
--prompt "a cute anime girl in school skirt uniform holding a gun \
with fantastic lighting fantastic composition \
in PlayStation5 octane render style" \
--negative-prompt "twisted face twisted body closeup view"
白い
AtCoder August 2022
2022-08-16
ACM
1157
ABC264 on 13rd
E. Blackout 2
Problem: https://atcoder.jp/contests/abc264/tasks/abc264_e
問題文
ある国には N 個の都市と M 個の発電所があります。これらを総称して地点と呼びます。
地点には 1,2,…,N+M の番号がつけられており、そのうち都市は地点 1,2,…,N で発電所は地点 N+1,N+2,…,N+M です。
この国には電線が E 本あり、電線 i ( 1≤i≤E ) は地点 Ui と地点 Vi を双方向に結びます。
また、ある都市に 電気が通っている とは、ある都市から電線をいくつか辿って少なくともひとつの発電所に辿り着くことができる状態を言います。
今、 Q 個のイベントが起こります。そのうち i ( 1≤i≤Q ) 番目のイベントでは電線 Xi が切れ、その電線を辿ることができなくなります。一
Review of Linear Algebra
2021-10-20
Math
336
Matrix
Basic Operations
Matrix Product (Dot Product)
Matrix Division
Matrix Multiplication (Hadamard Product)
Matrix Operations
Matrix Transpose
Matrix Inversion
Matrix Trace (the sum of the main diagonal elements)
Matrix Determinant
Matrix Rank (the maximum number of linearly independent columns)
Matrix Factorization (?)
LU Decomposition
QR Decomposition
Eigendecomposition
Singular Value Decomposition
Ref: Linear Algebra Cheat Sheet Python Code
Others:
Unitary Matrix
A matrix that satisfies
Minor Matrix
Adjugate Matrix
For square matrix A, , , where is the (i, j) minor of A.
Conjugate Transpose
, for complex number ,
Orthogonal Matrix
A matrix that satisfy
Matrix Product
Matrix product is produced by taking i-th row of the first matrix and j-th column of the second matrix and calculate dot product of the two vectors as the (i, j) element in the result matrix.
Given
then,
Matrix Division
Matrix Division makes use of the following property:
where
NLP with Deep Learning
2021-09-09
Machine Learning
332
Transformer
Attention is all you need - Arxiv
Feed Forward: Two fully connected layers (Linear) with a ReLU activation in between.
Multi-head Attention:
Attention
Attention - Qiita
Self-Attention
GPT (Generative Pre-Training)
GPT - paper
BERT (Bidirectional Encoder Representations from Transformers)
BERT - Arxiv
BERT Explained
Different from the Transformer (GPT) which is trained using only the left context, BERT uses bidirectional encoder which makes use of both the left and the right context. [MASK] is used to mask some of the words so that the model will not see the word itself indirectly.
Pre-training of BERT makes use of two strategies: MLM (Masked Language Model) and NSP (Next Sentence Prediction). The model is trained with both the strategies together.
As shown below, the input embeddings of BERT consists of the token embeddings, the segment embeddings, and the position embeddings. Note that a segment may consists of multiple sentences.
In MLM task,
HomeVR Dev Log
2020-11-09
Tech
589
Nov 04
WebVR requires HTTPS on Oculus Quest.
WebVR seems to require enabling Universal PR (Will cause some issues)
Universal PR supports ShaderGraph, which is good. But it does not support point light shadows, which is bad.
By default, Universal PR only enable shadows for the main light. Go to UniversalRenderPipelineAssset > Lighting > Additional Lights, enable Cast Shadows. You may also want to enable Shadows > Soft Shadows.
The PostProcessing Volume for Universal PR is Create > Volume > Global Volume. Some suggest creating a empty GameObject and add PostProcessingVolume to it. Do not work for me. (Maybe it is because the Volume Mask is set to Default)
Nov 09
Unity do not have Mirror support for build-in pipeline and URP. HDRP has planar reflection. To make planar reflection work in URP, you may use some tricks like reflection probe or simple reflection camera logics. But these only work for small planar reflection. For larger mirror, they are broken
Followers
2020-07-16
Articles
587
一开始我是被 PV 吸引了。有点意思,我想。女主有点酷;虽然 PV 完全意味不明,但是就是很戳。大概是因为色彩主题和几分前卫的内容。
第一集看下来,女主(似乎中文翻译应该是叫夏目?)果然很酷,而且穿衣也很潮。(虽然她那个画家朋友比女主还拽就是了。)而且女主手机,屏幕碎了都舍不得换,不得不说细节简直了。后来看到说是演员自己觉得这样的细节更符合女主的人设。天,秒粉了(不是)。而放在写这篇评论的现在,我的手机屏幕也碎了 n 久都还不想换,就更有共鸣了(笑)。
如果单看女主的线,其实剧情还是蛮套路的;但却不乏味。而这部剧的其实不止一个主角。摄影师奈良和她的朋友们也占了同等重要的戏份。这是一部以多名女性为主角,藉此反映社会现象和新文化的番剧。这部剧融合的文化元素可谓是前卫的大杂烩。男主是一名 Youtuber ;
Tic-Tac-Toe Online
2019-10-10
Tech
252
Tic-Tac-Toe Online Server
Base on the Tic-Tac-Toe Game of CS188, Berkeley, I develop an online version of Tic-Tac-Toe. Now your agent can play with my agent online!
I think it is a good way to check whether our agents are optimal or not.
My agent can beat random agents most of the time even if my agent is the second player.
Online Server Website: Tic-Tac-Toe Online
Download the attached client file from the moodle form and place it in the same directory of your solveTicTacToe.py (solveTicTacToe.py depends on util.py , so util.py is also needed).
Run this command:
$ python3 TicTacToeOLClient.py -u demo -n 3
And Enjoy!
Notice: You need to specify a username with "-u USERNAME". Don't use "demo" as your username cause it is forbidden.
Usage: TicTacToeOLClient.py [options]
Options
Variable
Description
-u
USERNAME
Username, must not be empty nor
Shenyang2019.icpc D Fish Eating Fruit
2019-09-18
ACM
419
The Question
Fish eating fruit on jisuanke
Given an undirected acyclic graph G, all possible path P in the graph, calculate:
The first taste
In the contest, a handsome foriegn teammate conviced me that this problem can be solve using LCA. I tried. And it did work, with the help of dijkstra.
My solution is to, first of all, run dijkstra, and get the distance between root node and every other nodes. Then, calculate the LCA for every two nodes. The desired result is:
It worked, but we got TLE for looping though all the nodes, which is .
The second trial
After the contest, I was told that this is a DP problem. You calculate the times an edge is accessed, times it with the weight, sum them up by the modulus of 3, you got the result.
This one, however, also got TLE. Oh, FISH!
The final solution
The reason why the second solution still can
R-CNN
2019-07-07
Computer Vision
2671
Overview
Regions with CNN features:
Efficient Graph Based Image Segmentation
use disjoint set to speed up merge operation
Selective Search
HOG (Histogram of Oriented Gradient)
Multiple criterions (color, texture, size, shape) to merge regions
AlexNet/VGG16
R-CNN
Notice that many descriptions are replicated from the orignal sources directly.
Some Fundermental Conceptions
Batch Size
Stochastic Gradient Descent. Batch Size = 1
Batch Gradient Descent. Batch Size = Size of Training Set
Mini-Batch Gradient Descent. 1 < Batch Size < Size of Training Set
Regularization
A regression model that uses L1 Regularization technique is called Lasso Regression and model which uses L2 is called Ridge Regression.
Ridge Regularization
Ridge regression adds "squared magnitude" of coefficient as penalty term to the loss function.
The first sum is an example of loss function.
Lasso Regularization
Lasso Regression (Least Absolute Shrinkage and Selection Operator) adds "absolute value of magnitude" of coefficient as penalty term to the loss function.
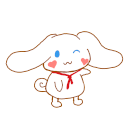